Going from Seed to Series A is the biggest leap in startup financing:
At Seed you won’t have much figured out — maybe just an idea or a prototype — so your first investors, customers, and hires expect a lot of rough edges. At Series A, you’ll actually have something — ideally early traction in a promising market — so you’ll need to get somewhat organised for fundraising.
In the funding environment of 2021–2022 you might have gotten away with some crazy stuff. But diligence has returned in 2023, and VCs are doing a lot more digging into your business and its numbers before making an investment.
There are many comprehensive lists online of items that you could include in a data room. But the truth is that VCs will ignore the vast majority of these items, and only a few key data points will actually move the needle.
As a founder, your goal is to create the “minimum-viable-data room”: spending the least time possible, to raise the necessary $, on the best terms.
Before we dive into the data room, there are 3 important things to bear in mind:
- The quality of your business and metrics is much more important than the quality of your data room — don’t miss the forest for the trees!
- The better your metrics, the less detail you’ll be asked to go into. At the extreme end of the spectrum (even for 2021), Deel raised $629 million without creating a pitch deck. While this isn’t true for almost everyone else, it’s still important to remember that better numbers = less data required.
- If you’ve never missed a flight, you’ve spent too much time in airports, and if you never get asked to send more information, you probably over-supplied! (You’re probably not helping the investors find the signal that you’d like them to).
We’ve assembled this list for founders and early finance hires at a typical B2B SaaS company, with useful generalisations for all startups.
The Minimum Viable Data Room
Revenue Analysis
Traditional methods of analysing a business (a discounted cash flow model) aren’t helpful at Series A. At this early stage, investors are trying to understand something more fundamental: do customers like your product? How much do they like it? Do you have product-market fit?
If only datapoint could answer these questions, it would be a revenue analysis. The rest of an investor’s analysis flows from understanding this, and all the other datapoints you provide will only serve to clarify or disqualify your revenue analysis.
Here’s the best format for a revenue analysis:
If you’ve got the revenue data in a time-series format on another page in the same Google Sheets file, that’s useful too. In terms of information density, this is the most valuable document for a Series A investor.
From this data source, they can infer so much about the company: they can see the buildout of customers, and see how much people like the product. Many of the calculations for your pitch deck are done from this data. Here’s a Causal template which you can put your revenue into to do these calculations. (And here’s an explainer on putting time-series data into Causal.)
Some important things to keep in mind:
- In time-series data, monthly increments are best.
- Investors like raw data to do their own analysis. (Don’t require them to reverse engineer the numbers they want from your own weird metrics!)
Usage Data
Usage data shows whether your product actually delivers after a customer buys it. It acts as a leading indicator of revenue expansion (if there’s high usage) or churn (if there’s low usage).
The importance and format of usage data will vary company-by-company, and so it’s hard to demonstrate what usage data should look like.
A key consideration for all businesses, however, is the congruency between claims in their pitch and the evidence of their usage data. In the case of B2B software, a PLG company should be able to show usage metrics (and an increasing ACV in their revenue analysis) to qualify this.
For a B2B SaaS Company, investors will want to know these high-level metrics:
- DAUs/WAUs/MAUs, and proportion of customers that are in each bucket
- Seats per customer
- Time spent in the product (per day/week/month, depending on your product)
Sales Funnel
Depending on the development of your revenue analysis, you might also want include a sales funnel. This is a leading indicator of what your revenue analysis will look like in a few months time, and will help investors understand the quality of your go-to-market motion.
The important information for the sales funnel is:
Important thing to keep in mind:
- Give investors a % conversion rate for each defined stage of the funnel. If you want investors to attribute prospects in the funnel to future revenue, they'll need to do an expected value calculation, using the contract size and probability of conversion.
Basic P&L
A Profit-and-Loss statement (P&L) is a standardised table of data that gives investors a high-level view of your company’s financial health, without needing to know the ins-and-outs of your business. It shows your Revenue (”how much money do you make?”) and Costs (”how much money do you spend?”) broken out into relevant categories (e.g. Revenue by Product, Marketing Expenses vs Engineering Expenses).
Compared to 2021–22, ‘growth’ is no longer the be-all and end-all — investors will also look at efficiency metrics like Burn (”how much money do you lose per month?”) and Margin (”how much do you have to spend to generate $1 of revenue?”).
Spending data contextualises revenue numbers. It can either make revenue numbers look good, if the company has particularly few employees, or spends little on sales and marketing (S&M). But it might equally disqualify your revenue numbers: “It’s great that you added $X revenue, but your S&M spend of $Y was driving this, so it’s not as impressive.” Causal’s Startup Suite has free templates for creating a P&L model and calculating monthly burn.
The metrics which an investor will most commonly want to know from a Series A company’s P&L are:
A common error is to confuse COGS and OPEX. Putting COGS in OPEX can make the gross margin look artificially better. The COGS are spending which relate directly to delivering the product (for example, the server costs and payment processing), whereas the OPEX costs are indirectly incurred from running the business (e.g. salaries, and renting office space).
In these financial conditions, spending is not inherently bad but investors want to understand how it contributes to growing the top line. Burn Multiple is a good way of assessing this. It measures capital efficiency: for each $ of spending, how many $s does the company add to their revenue (top line). The formula is:
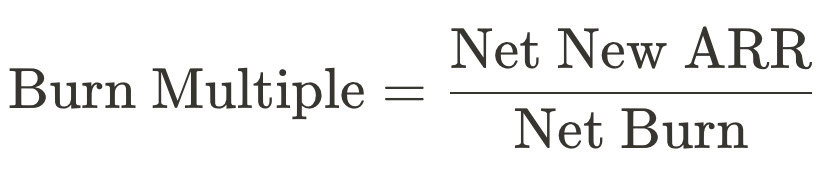
Bonus: The 1 Mistake to Avoid
The #1 mistake to avoid is unreasonable market sizing — making very large claims about market size, without a combined specific insight, is a negative signal.
At Series A, investors will use the TAM to price the business insofar as the TAM is actually addressable. (This too can be extrapolated from revenue analysis!) For example, if you have a customer paying $50k/year, and there’s 1000 companies in the world of the same archetype (as in, that can use the product in the same way), the investors can reasonably say there’s a $50 million/year market for your product, or at least this is a useful approximation.
There’s a general tendency to overestimate the extent to which you might be able to upsell or create new product lines. For a Series A company, expanding ACV is a positive signal, but at Series A, when investors invest, they’re aren’t pricing the company on (for example) the entire market size of financial services. Instead, the customer that you’ve already addressed can be extrapolated to create a “base case” for how many customers you might reasonably address. (Everyone wants to be a platform!)
Conclusion
Every startup has its unique elements, and it's not the case that every data room will look the same. Whatever metrics you include, you're trying to answer the fundamental questions: do customers like your product? How much do they like it? And do you have product-market fit?
The factors discussed in this post: a revenue analysis, usage data, a sales funnel, and a basic P&L are striving towards answering these questions, at the most basic level. Investors appreciate sticking to answering these fundamental questions in a basic way, because they're looking to make an apples-to-apples comparison to their hurdle rate. (Too much innovation in metrics tends to imply smoke and mirrors!)
Appendix: Future Spending Plans
It's worth noting that you should expect to be asked about your future spending plans. The rationale of capital efficiency applies in equal measure to past spending and future spending. Investors want to understand how you’re planning to spend in the future and how this will contribute to the top line. Causal has a suite of pre-built models (revenue forecast, cohort models, P&L) that will help you share more accurate plans with potential investors. For example, if enterprise sales is important to your company, you’ll need an sales “ramp-up” model which explains:
- The number of SDR’s you’ll need to hit your targets
- The productivity these people will need to achieve to hit their targets
- How fast they’ll be able to ramp up to reaching these targets
Using Causal allows you to communicate the future potential of your company with interactive dashboards, which account for uncertainty and variance, and allow people to input their own assumptions without the risk of messing with the underlying model.